Artificial intelligence has seen significant progress in enhancing its effectiveness and strength. However, reinforcement learning has taken AI to a whole new level. By incorporating real-time feedback loops in AI algorithms, reinforcement learning has opened up possibilities to push the limits of AI’s capabilities and usher it into a new era of advancement.Ā
This advanced AI technology is revolutionizing our understanding and application of artificial intelligence by integrating complex learning environments, adaptive algorithms, and reward systems. The article below delves deeper into this groundbreaking technology’s current uses and potential future applications.
So, let’s get started.
What is Reinforcement Learning?
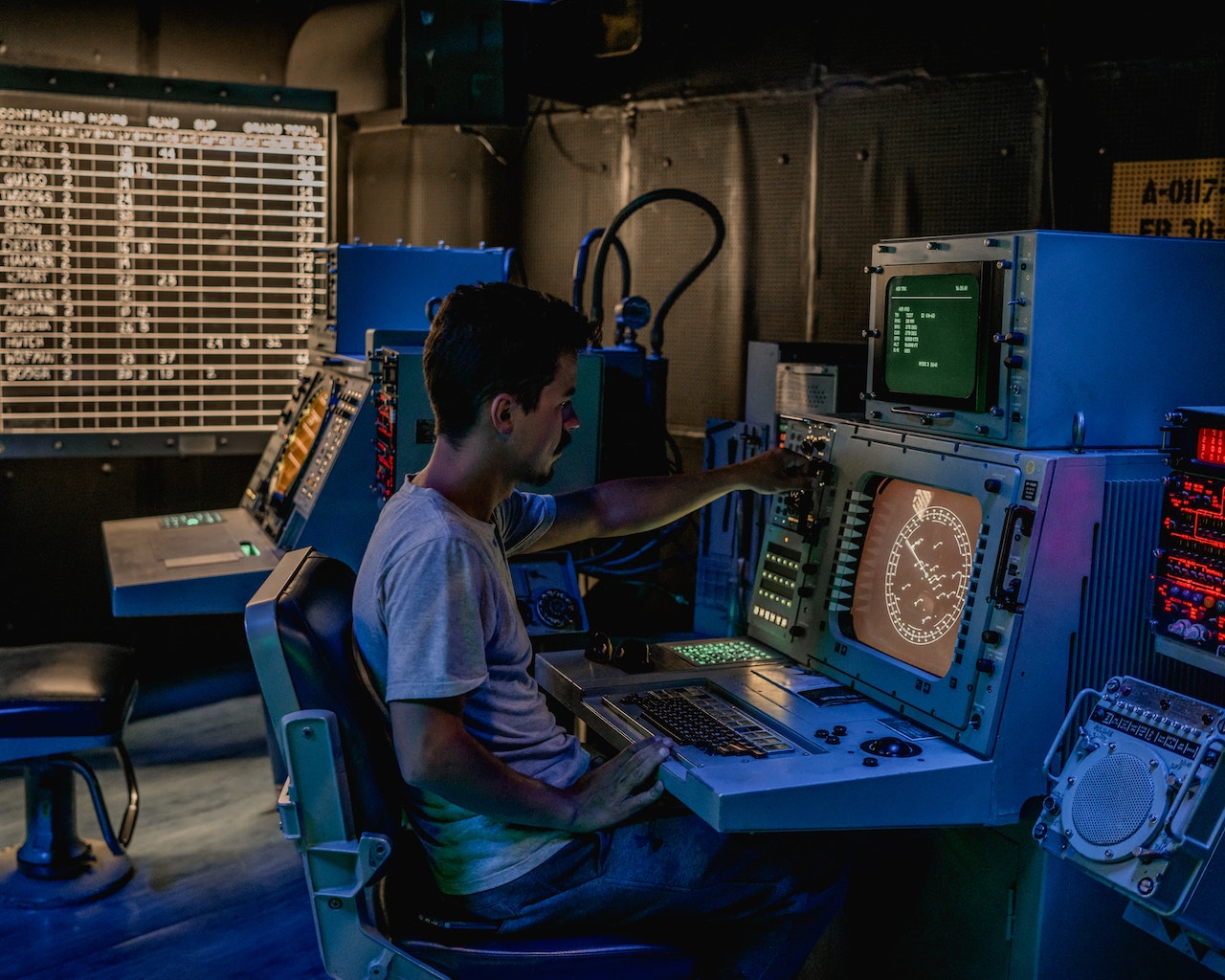
Reinforcement Learning is a sort of machine learning which enables an agent to learn from its environment by testing different actions. The agent gets an award for executing specific actions, and these rewards motivate the agent to know which actions provide the best outcome for a given situation.Ā
Reinforcement learning has given the power to machines to solve complex problems like playing chess or navigating a maze with exceptional accuracy. Reinforcement Learning jobs are also becoming much more popular as technology is becoming increasingly used in various fields.
Applications of Reinforcement LearningĀ
Here are some key areas where RL has made significant contributions:
- Robotics: RL has enabled robots to learn & adapt to their environment, allowing them to perform previously challenging or impossible tasks. Robots can learn how to grasp objects, navigate in dynamic environments, and even manipulate complex objects through RL algorithms. This has implications for manufacturing, healthcare, and logistics industries, where autonomous robots can assist with tasks that require dexterity and decision-making.
- Game playing: RL has achieved remarkable success in game playing, surpassing human performance in games like chess, Go, and Atari games. By training AI agents through RL algorithms, they can learn optimal strategies, explore different tactics, and master complex game dynamics. The application of RL in game playing has not only demonstrated the capabilities of AI but also provided insights into problem-solving and strategic thinking.
- Autonomous vehicles: RL plays a crucial role in developing autonomous vehicles. RL algorithms enable vehicles to learn to navigate complex traffic scenarios, make decisions in real time, and optimize energy efficiency. By training through simulation and real-world interaction, autonomous vehicles can continually improve their driving skills, ensuring safety and enhancing transportation efficiency.
- Recommendation systems: RL is used to personalize user recommendations in various domains, such as e-commerce, streaming platforms, and online advertising. RL algorithms can optimize recommendations to maximize user satisfaction and engagement by considering user preferences, feedback, and interaction history. This enables businesses to deliver targeted and relevant content, enhancing the overall user experience.
- Healthcare: RL has promising applications in healthcare, ranging from personalized treatment plans to medical image analysis. RL algorithms can optimize treatment strategies for patients with chronic diseases, considering individual characteristics and responses to therapy. In medical imaging, RL can assist in automated diagnosis, detecting abnormalities, and aiding in interpreting medical images.
- Natural Language Processing: RL has been instrumental in advancing natural language processing tasks, such as machine translation, dialogue systems, and language generation. RL-based models can learn to generate human-like responses, understand and respond to user queries, and carry on meaningful conversations. This has significant implications for customer service, virtual assistants, and content generation.
4 Ways Reinforcement Learning is advancing AI
1. Improving adaptability
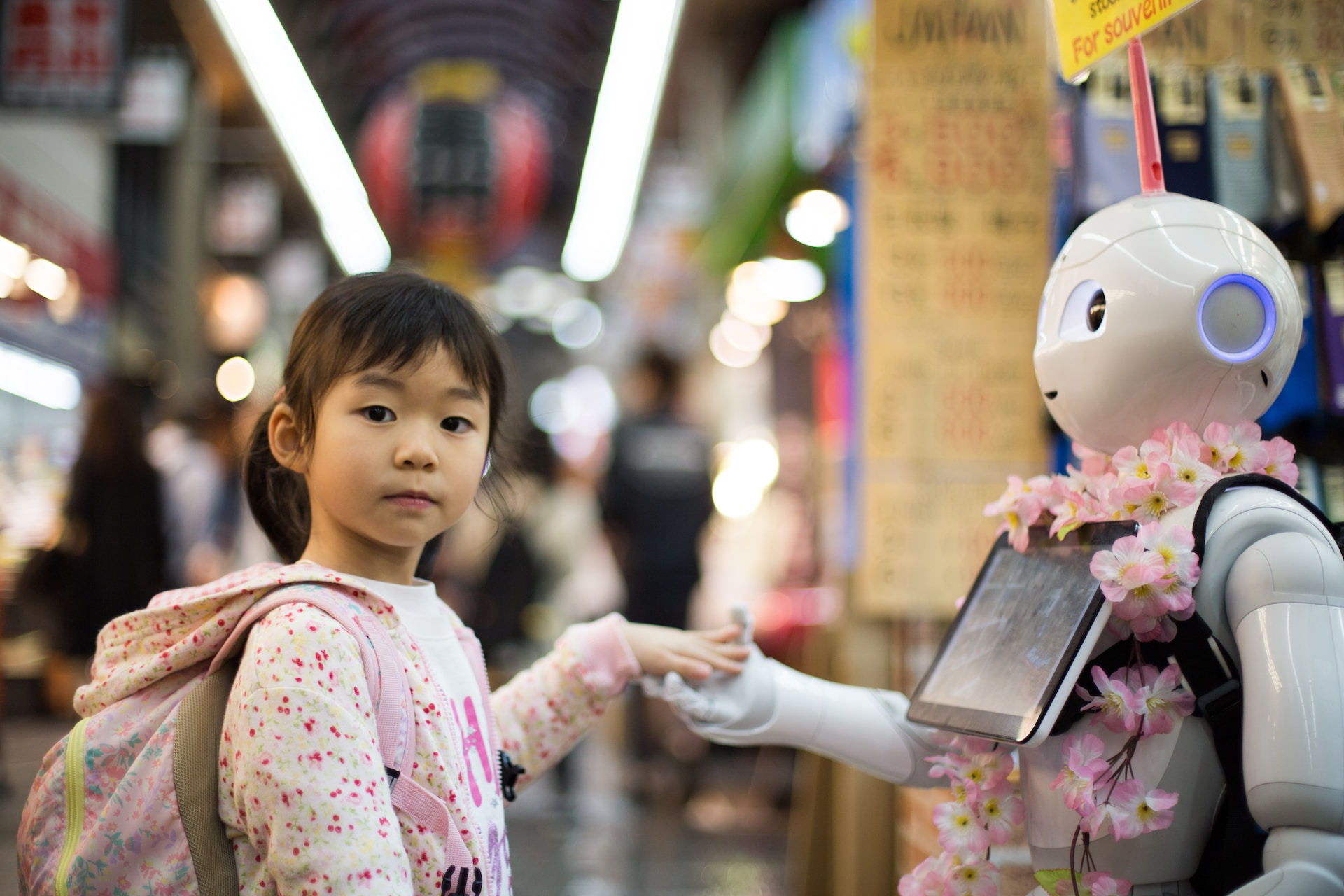
Reinforcement Learning (RL) advances AI by enhancing its adaptability to different environments and situations. RL agents can learn and adjust their behavior based on feedback from their interactions with the environment. This adaptability allows AI systems to tackle new and evolving challenges, as they can continuously learn and update their strategies to achieve better performance.
2. Enhancing decision-making abilities
RL is empowering AI systems with enhanced decision-making capabilities. By learning through trial and error, RL agents can also understand the consequences of their actions and make informed choices. The feedback in the form of rewards or punishments guides the agents to learn optimal strategies over time.Ā
3. More realistic representation of solutions
Reinforcement Learning enables AI to generate more realistic representations of solutions to complex problems. RL agents can learn from diverse experiences, exploring different paths and learning from both successful and unsuccessful outcomes. This comprehensive learning process results in AI systems that can provide more accurate and nuanced solutions, considering various factors and dependencies.Ā
4. Training complex behaviors
One of the remarkable aspects of RL is its ability to train AI systems in complex behaviors. RL algorithms can handle situations where traditional programming approaches are limited or impractical. By allowing AI agents to learn from their interactions with the environment, RL can train them to exhibit sophisticated behaviors that were previously challenging to program explicitly.
The bottom line
Reinforcement Learning (RL) is a game-changer in Artificial Intelligence (AI), continuously pushing the boundaries and advancing the capabilities of AI systems. RL empowers AI agents to adapt, make decisions, and achieve complex tasks in dynamic environments through its unique learning paradigm.